by Margaret Golz, Richard Wysk, Russell King. Colin Nolan-Cherry, & Stephen Bryant (North Carolina State University)
As presented at the 2018 Winter Simulation Conference
Medical device companies have a growing interest in additive manufacturing (AM) for orthopedic implant production. AM provides an opportunity for economically feasible customized implants to supplement the current traditionally-manufactured, standard-sized prosthesis. In this paper, a simulation model is developed to analyze the supply chain of custom hip stem production using AM. A test case is implemented into the simulation model to determine the supply chain’s responses in key performance indices, such as production lead time of a uniquely customized prosthesis and resource utilization, to resource level variations based on current state of the practice for additive and subtractive manufacturing. The method of this research was to use a simulation focused on measuring the performance of the supply chain for hip stem implants in order to determine supply chain resource requirements.
Introduction and motivation
Arthroplasty, commonly called joint replacement, uses standard-sized prostheses that are pieced together to form a mechanical joint. Joint replacements become necessary when a patient suffers significant impairment from constant, severe joint pain and conservative treatment has been exhausted. Arthroplasty operations are relatively safe and can relieve pain as well as restore like-normal mobility. Due to significant stress and strain on the body through daily activities, implants will wear down and can fail; the 25-year survival rate of a hip implant is over 80% (Shan et al. 2014). When an implant fails, a much more costly, difficult, and riskier revision operation is necessary. Over a million total joint arthroplasty surgeries are performed annually in the U.S.; total hip arthroplasty (THA) comprise approximately a third of these (OrthoInfo 2017).
Figure 1 shows the three parts of a hip replacement implant: the femoral (hip) stem (inserted into the medullary cavity in the femur), acetabular component (replaces the socket in the pelvic region), and the femoral head (articulates the femoral stem to the acetabular component while allowing normal hip motion); each part comes in several sizes. Currently, traditional manufacturing methods are typically used to produce standard hip implant sizes and geometries for all patients. Conventional methods for manufacturing prosthesis hip stems depend on the type of material in use. Forging or investment casting are the typical procedures for medical device metals such as titanium, cobalt chromium, and stainless steel. After the basic geometry is shaped, rough machining, polishing, and coating complete the process (Zhang et al. 2009). Standard hip stems are not custom-made for individual patient use, therefore, not all prosthesis hip stems fit perfectly or are guaranteed to work well.
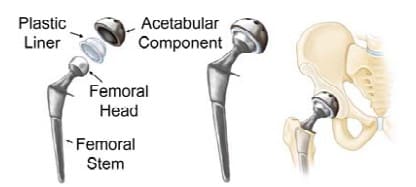
The medical field has grown increasingly interested in alternatives to standard medical implant sizes because of the anticipated benefits. Through customization, an implant can be adapted to accommodate the patient’s specific anatomy including bend stiffness (directly connected with aseptic loosening), leg length discrepancy, and highly deformed femurs (Cronskär et al. 2013). Aseptic loosening, the loosening of a prosthesis due to wear or failure of osseointegration, is a major cause of revision surgery. Custom hip implants often reduce the amount of bone removal necessary for aligning the hip stem during surgery. It is important to preserve as much bone as possible in case revision surgery is needed later. Surgery using custom hip implants should be relatively straightforward because the implant would better match the bone geometry of the patient, although it’s likely standard bone cuts would not be used. A simple fit also could lead to shorter surgery times and should reduce the likelihood of aseptic loosening (Harrysson et al. 2007). Research is in the early stages, but a small number of studies suggest customized prostheses have the potential to decrease the pain and suffering, risk of infection, and therapy time for the patient. It could possibly increase the life of the medical implant, postponing or eliminating revision surgery.
Additive manufacturing, also called rapid prototyping or 3D printing, has shown the capability to create custom implants from patient computer tomography (CT) scans; it has the ability to create unique, complex geometries relatively easily because it builds parts by laying down material a single cross section at a time. AM presents the opportunity to economically customize medical implants to better fit the patient’s anatomical geometry and activity level needs. A specific type of AM, Electron Beam Melting (EBM), is advantageous in the creation of medical devices because it can be used to produce prostheses using a titanium alloy that is already FDA-approved. Further, the surface finish of the titanium alloy Ti6Al4V when fabricated using EBM technology is biocompatible and has equivalent or possibly increased osseointegration compared to traditional implants (Haslauer et al. 2010; Thomsen et al. 2008). Parts built by EBM have less material waste relative to their standard part production by traditional manufacturing methods (Sing et al. 2015). EBM is currently in use for production of standard orthopedic implants (trabecular structures specifically) with CE-certification and FDA-approval (Arcam AB 2018).
There is a selected number of companies that are producing acetabular cups using Ti6Al4V with Arcam’s EBM machine. One such company, Adler Ortho Group, began producing CE-certified hip implants in 2007 (Arcam EBM 2018). The acetabular cups are only stock size and as of early 2014, over 90,000 implants have been produced by Adler Ortho and other companies; 40,000 of these EBM manufactured acetabular cups have been implanted. Also as of 2014, approximately 20 distinct medical devices fabricated using AM have gained FDA clearance for implantation (Gibson et al. 2015).
The continuous enhancement of AM has shown that it could potentially meet a portion of the current demand with custom implants or meet full market demand with a combination of standard and custom devices. For a medical device company, the AM technology opens the door to an unfamiliar mass production method; research is necessary to predict the required resources and limitations of large-scale production of custom implants. Modeling the supply chain can manifest upstream and downstream behaviors for the manufacturer. Supply chain simulation is a valuable tool that can provide insights of the supply and demand patterns as well as an evaluation of system resources. This research is focused on the development of a supply chain model of patient specific hip stems, model implementation into supply chain simulation software, and sensitivity analysis on resource levels.
Background
There have been multiple studies exploring how AM could be used for orthopedic medical implants. Two previous studies have used CAD modeling to design a custom femoral component of a knee replacement from a patient’s CT scan (Harrysson et al. 2007, Harrysson et al. 2003). In one study, finite element analysis (FEA) was performed on the custom and conventional CAD model files to evaluate stress distribution. The results showed several promising advantages of custom implants to the conventional ones: more even stress distribution decreasing the risk of premature loosening, decreased need for surgical interventions and filler components (due to a better fit), and about 40% less bone removal. The group determined that some of the disadvantages could be mitigated by the use of AM for component fabrication (Harrysson et al. 2007).
sadvantages could be mitigated by the use of AM for component fabrication (Harrysson et al. 2007). The second study created a custom femoral component of a canine knee implant using EBM and compared the creation time to traditional investment casting of the same custom part. The traditional method took more than three times as long as the EBM process to produce the custom implant. The component for the EBM machine was designed from a CT scan of the patient with contributions from orthopedic surgeons; file preparation for fabrication took 1.5 hours. The custom design was created out of Ti6Al4V powder, a material that has been FDA-approved for implantation into patients for particular medical devices created using AM. The group determined that EBM technology can be used to fabricate custom medical implants and design porous surfaces directly into the component (Harrysson et al. 2003).
Thomsen et al. (2008) performed an in vivo bone ingrowth analysis of Ti6Al4V EBM implants and found no appreciable difference in tissue response from polished, traditionally manufactured titanium alloy implants. The study also found osseointegration on the structural level. Prior to implantation, the parts were ultrasonically cleaned for 25 minutes. After washing, the implants were steam autoclaved in sterile bags.
hip stem prosthesis. The group fabricated 7 hip stem implants using an Arcam A2 EBM machine and machined the same set of implants for cost comparison. The implants were oriented vertically during file preparation in order to obtain the best surface finish. The study further simulated a build with 14 custom implants to observe the processing time for full capacity of the EBM machine. The simulation machine processing time for 7 and 14 implants was 36 hours and 38 hours respectively. The actual processing time for the 7 implant print approximately matched the simulation time; there was a cool-down time of 8 hours after the completion of the build. Wasted material from the build measured 24% of the total weight of the implants; the loss of material from the EBM-fabricated parts was 35% less than the conventional method. The study reported EBM file preparation (CAD file modification, support generation, and compilation) lasted about 40 minutes. Before printing, the EBM machine took just over an hour to warm up and draw the vacuum in the chamber. After printing, the operators had to remove excess powder and clean the machine/implants which also took approximately one hour. Following EBM printing, subtractive manufacturing with CNC machines contributed an additional 2 minutes per implant for milling and 1.5 hours per implant for grinding and polishing. The group concluded that AM custom implants are commercially viable and are economically advantageous over machined ones (Cronskär et al. 2013).
Research completed by Shouche (2016) presented a supply chain model for custom hip stems created with AM. Supply chain operations reference models were created for custom and traditional hip stems; the research focused on the comparison of the two models using performance metrics. The custom hip stem model was implemented using Arena simulation software and is shown in Figure 2; it was the inspiration for continued research on the topic. The model simulates the process of each patient’s order starting from the physician’s diagnosis and finishing with the implant delivery to the hospital or discarding the implant if the part is insufficient. This process includes CAD modeling, EBM fabrication, and CNC machining to machine finish the implant. Resource requirements to satisfy an annual demand of 166,000 implants (approximately half of the THA performed annually) were determined through simulation; the system required 1,190 medical engineers to complete the CAD modeling and 110 EBM machines (both at 65% utilization). The study further determined that the metal powder to fabricate the hip stems and the number of EBM machines were the most sensitive to volume production change (Shouche 2016).
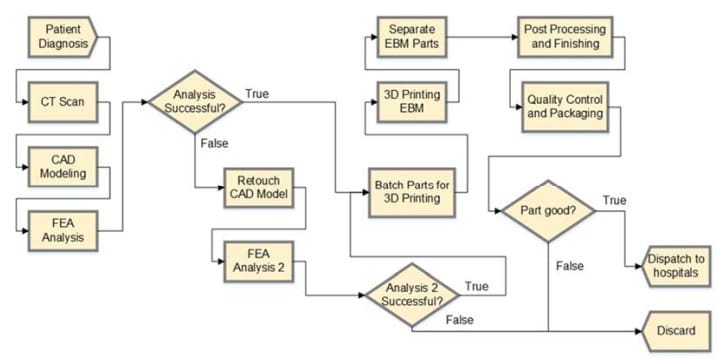